Comprehensive Integration of Single Cell Data
The comprehensive integration of single-cell data represents a transformative approach in modern biological research. By leveraging advanced computational techniques, scientists can now unravel the intricate complexities of cellular heterogeneity and function. This article delves into the methodologies and applications of integrating single-cell data, highlighting its potential to revolutionize our understanding of cellular processes and disease mechanisms.
Introduction
Single-cell RNA sequencing (scRNA-seq) has revolutionized our understanding of cellular heterogeneity by allowing researchers to examine gene expression at an unprecedented resolution. However, integrating single-cell data from different sources and experiments remains a significant challenge due to variations in technology, batch effects, and biological diversity. Comprehensive integration of single-cell data is essential for drawing meaningful conclusions and advancing our knowledge in various biological and medical fields.
- Combining data from multiple scRNA-seq studies to increase statistical power and robustness.
- Addressing batch effects and technical variations that can obscure biological signals.
- Utilizing advanced computational tools and algorithms for effective data integration.
- Leveraging services like ApiX-Drive to automate and streamline the integration process.
By employing sophisticated integration techniques and leveraging automation tools like ApiX-Drive, researchers can more effectively harmonize datasets, uncover new insights, and accelerate discoveries. This comprehensive approach not only enhances the reliability of single-cell analyses but also facilitates collaborative efforts across different laboratories and research institutions.
Computational Methods for Single Cell Data Integration
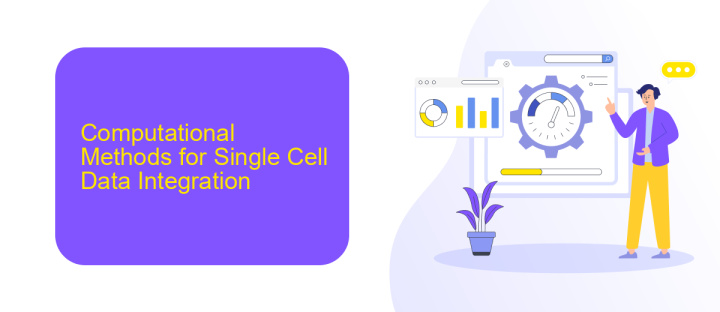
Integrating single-cell data from diverse sources requires sophisticated computational methods to ensure accurate and meaningful analysis. One approach involves the use of alignment algorithms that match cells across datasets based on their gene expression profiles. Techniques such as canonical correlation analysis (CCA) and mutual nearest neighbors (MNN) are commonly employed to identify and correct batch effects, ensuring that data from different experiments can be compared directly. These methods help in creating a unified dataset that can be used for downstream analysis, such as identifying cell types or inferring developmental trajectories.
Another crucial aspect is the use of scalable and efficient software tools that can handle the large volumes of data generated in single-cell experiments. Platforms like ApiX-Drive can facilitate the integration process by automating data synchronization between different bioinformatics tools and databases. This reduces the manual effort required and minimizes the risk of errors. Additionally, machine learning algorithms are increasingly being used to enhance the integration process, offering improved accuracy and the ability to uncover hidden patterns in the data. These computational methods collectively ensure that researchers can derive robust and reproducible insights from single-cell datasets.
Biological Applications of Single Cell Data Integration
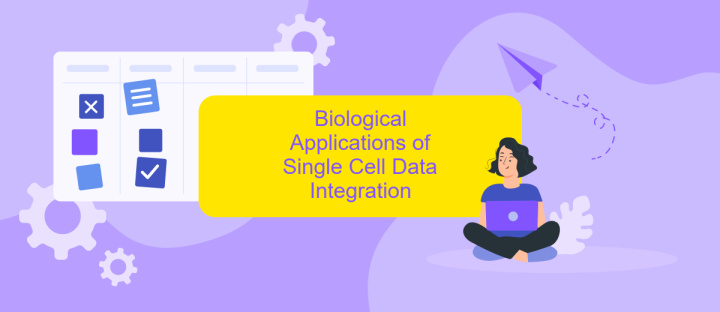
Single cell data integration has revolutionized our understanding of cellular heterogeneity and tissue complexity. By combining data from multiple single-cell experiments, researchers can gain a comprehensive view of cellular functions and interactions, which is crucial for various biological applications.
- Identifying Cell Types: Integration helps in accurately identifying and characterizing different cell types within a tissue, providing insights into cellular diversity.
- Tracing Lineage Relationships: By integrating single-cell data, scientists can trace the lineage and developmental trajectories of cells, which is essential for understanding development and disease progression.
- Discovering Biomarkers: Integrated single-cell data can reveal novel biomarkers for diseases, aiding in early diagnosis and personalized treatment strategies.
- Understanding Cell-Cell Interactions: Integration allows for the analysis of cell-cell communication networks, shedding light on how cells interact within their microenvironment.
Tools and services like ApiX-Drive facilitate the seamless integration of single-cell data from various sources, automating the data merging process and ensuring high-quality results. This enables researchers to focus more on biological insights rather than technical challenges, accelerating discoveries in fields such as immunology, oncology, and regenerative medicine.
Challenges and Future Directions
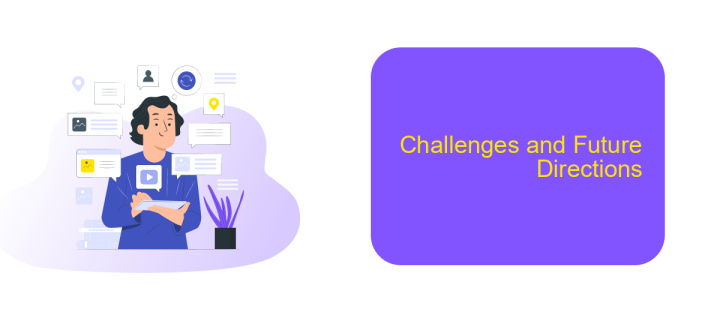
Integrating single-cell data from various sources presents significant challenges, primarily due to the heterogeneity of data types and the complexity of biological systems. Ensuring data consistency and accuracy requires robust computational methods and standardized protocols.
Another critical challenge is the scalability of data integration processes. As the volume of single-cell data grows exponentially, traditional methods may become inefficient, necessitating the development of more advanced algorithms and computational resources.
- Data heterogeneity and standardization
- Scalability of integration methods
- Ensuring data accuracy and consistency
- Advanced computational resources
Future directions involve enhancing integration techniques through machine learning and artificial intelligence. Tools like ApiX-Drive can facilitate seamless integration by automating data workflows and ensuring compatibility between different data sources. Continued collaboration between computational biologists and data scientists will be crucial in overcoming these challenges and advancing the field.
- Automate the work of an online store or landing
- Empower through integration
- Don't spend money on programmers and integrators
- Save time by automating routine tasks
Conclusions
The comprehensive integration of single cell data has revolutionized our understanding of cellular heterogeneity and function. By leveraging advanced computational tools and methodologies, researchers can now dissect complex biological systems at an unprecedented resolution. This integration not only facilitates the identification of novel cell types and states but also enhances our ability to map cellular trajectories and interactions in various biological contexts.
Moreover, the use of platforms like ApiX-Drive has streamlined the process of data integration, enabling seamless connectivity between diverse data sources and analytical tools. This has significantly reduced the time and effort required for data preprocessing and integration, allowing researchers to focus more on data interpretation and hypothesis generation. As we continue to refine these integrative approaches, the potential for groundbreaking discoveries in single cell biology remains vast, promising to unlock new insights into health and disease.
FAQ
What is single cell data integration?
Why is integrating single cell data important?
What are the common challenges in single cell data integration?
How can automation tools help in single cell data integration?
What are the best practices for integrating single cell data?
Strive to take your business to the next level, achieve your goals faster and more efficiently? Apix-Drive is your reliable assistant for these tasks. An online service and application connector will help you automate key business processes and get rid of the routine. You and your employees will free up time for important core tasks. Try Apix-Drive features for free to see the effectiveness of the online connector for yourself.