Difference Between ETL and Big Data
Understanding the difference between ETL (Extract, Transform, Load) and Big Data is crucial for modern data management and analytics. ETL is a traditional process used for data integration, while Big Data encompasses a broader spectrum of technologies and methodologies for handling massive volumes of diverse data. This article explores their key distinctions, use cases, and how they complement each other in today's data-driven world.
Introduction
In today's data-driven world, understanding the differences between ETL (Extract, Transform, Load) and Big Data is crucial for businesses aiming to leverage their data effectively. Both ETL and Big Data play significant roles in data management, yet they serve different purposes and are suited for different types of tasks.
- ETL: A traditional process used for data integration, moving data from various sources into a centralized data warehouse.
- Big Data: Refers to large volumes of diverse data that require advanced tools and techniques for storage, processing, and analysis.
While ETL focuses on structured data and predefined workflows, Big Data encompasses a broader range of data types and real-time processing capabilities. Tools like ApiX-Drive can streamline the integration process, making it easier to connect various data sources and automate workflows. Understanding these differences is essential for businesses to choose the right approach for their data strategy and achieve optimal results.
ETL Overview
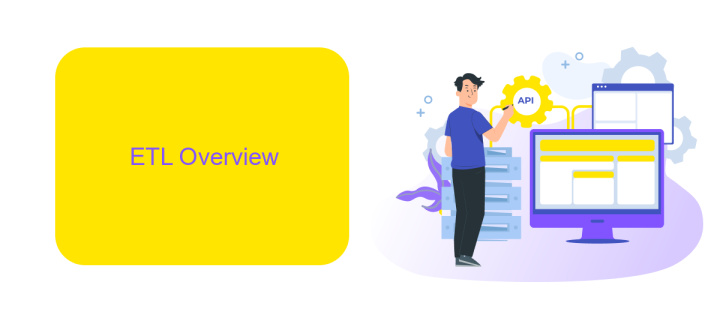
ETL, which stands for Extract, Transform, Load, is a data integration process that involves extracting data from various sources, transforming it into a suitable format, and then loading it into a target database or data warehouse. This process is crucial for consolidating data from disparate sources, ensuring that it is clean, accurate, and ready for analysis. ETL enables organizations to make informed decisions by providing a unified view of their data, facilitating better business intelligence and reporting.
Modern ETL tools and services, such as ApiX-Drive, simplify the integration process by providing pre-built connectors and an intuitive interface. ApiX-Drive allows users to automate data extraction from multiple sources, apply necessary transformations, and load the data into their preferred storage solutions without extensive coding. This not only saves time but also reduces the risk of errors, making data integration more efficient and reliable. By leveraging such tools, organizations can streamline their ETL workflows and focus on deriving actionable insights from their data.
Big Data Overview
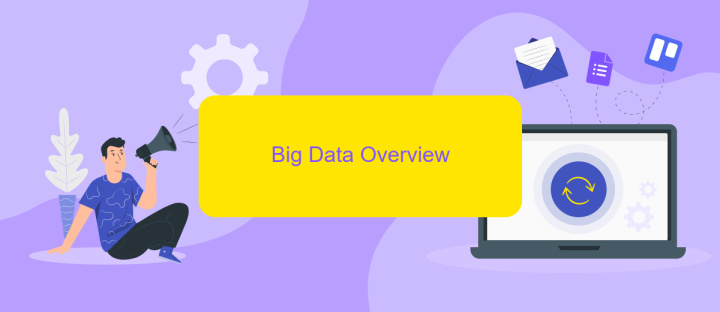
Big Data refers to the vast volumes of structured and unstructured data that inundate businesses on a daily basis. This data is so large and complex that traditional data processing software cannot manage it effectively. The importance of Big Data lies in its ability to provide insights that can drive better decision-making and strategic business moves.
- Volume: The sheer amount of data generated every second is staggering.
- Velocity: The speed at which new data is generated and processed.
- Variety: The different types of data, from text to images and videos.
- Veracity: The quality and accuracy of the data.
- Value: The potential insights and benefits derived from analyzing the data.
Managing Big Data often requires sophisticated tools and platforms. Services like ApiX-Drive can facilitate the integration of various data sources, making it easier to collect, process, and analyze Big Data efficiently. By automating data workflows and ensuring seamless integration, businesses can harness the full potential of their data assets.
Comparison of ETL and Big Data
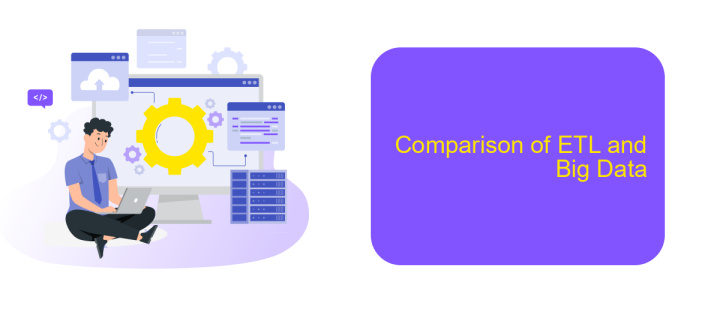
ETL (Extract, Transform, Load) and Big Data are two crucial concepts in data management, each serving distinct purposes. ETL is a process used to integrate data from multiple sources, transform it into a desired format, and load it into a target database or data warehouse. On the other hand, Big Data refers to the vast volumes of structured and unstructured data that require advanced techniques for storage, processing, and analysis.
While ETL focuses on data integration and transformation, Big Data emphasizes the management and analysis of large datasets. ETL is typically used in traditional data warehousing environments, whereas Big Data technologies are employed for handling massive datasets that exceed the capabilities of conventional databases.
- ETL: Primarily used for data integration and transformation.
- Big Data: Focuses on managing and analyzing large datasets.
- ETL: Suitable for structured data in data warehouses.
- Big Data: Handles both structured and unstructured data.
For businesses looking to streamline their data integration processes, services like ApiX-Drive can be invaluable. ApiX-Drive offers automated data transfer and integration solutions, making it easier to manage ETL processes and integrate various data sources efficiently. This can significantly enhance the overall data management strategy, especially when dealing with Big Data.
Conclusion
In conclusion, ETL (Extract, Transform, Load) and Big Data are both essential components in the data management landscape, but they serve different purposes. ETL is primarily focused on the structured and systematic processing of data from various sources into a unified format for analysis and reporting. It is a tried-and-true method for handling traditional data warehousing tasks and ensuring data quality and consistency.
On the other hand, Big Data encompasses a broader range of data types and processing techniques, often dealing with massive volumes of unstructured or semi-structured data. It leverages advanced analytics, machine learning, and real-time processing to derive insights from complex datasets. Tools like ApiX-Drive can facilitate the integration of ETL processes with Big Data platforms, enabling seamless data flow and enhancing the overall efficiency of data operations. Understanding the distinct roles and capabilities of ETL and Big Data can help organizations make informed decisions about their data strategy and technology investments.
FAQ
What is the main difference between ETL and Big Data?
Can ETL be used in Big Data environments?
What are some common tools for ETL and Big Data processing?
How do ETL processes handle real-time data in Big Data scenarios?
Why is data transformation important in both ETL and Big Data?
Do you want to achieve your goals in business, career and life faster and better? Do it with ApiX-Drive – a tool that will remove a significant part of the routine from workflows and free up additional time to achieve your goals. Test the capabilities of Apix-Drive for free – see for yourself the effectiveness of the tool.