Incorporating Uncertainty in Data Management and Integration
In today's data-driven world, managing and integrating vast amounts of information is increasingly complex. Incorporating uncertainty into data management and integration processes is crucial for enhancing decision-making accuracy and reliability. This article explores the methodologies, challenges, and benefits of addressing uncertainty, providing insights into how businesses and organizations can optimize their data strategies in an ever-evolving landscape.
Introduction
In the modern era of big data, managing and integrating vast amounts of information from diverse sources have become increasingly complex. One of the significant challenges faced by data scientists and engineers is the inherent uncertainty present in data. This uncertainty can stem from various factors, including data quality issues, incomplete data, and the dynamic nature of data sources.
- Data Quality Issues: Inconsistent and erroneous data can lead to unreliable insights.
- Incomplete Data: Missing values and gaps can hinder comprehensive analysis.
- Dynamic Data Sources: Continuously evolving data sources require adaptable integration strategies.
Addressing these challenges necessitates robust frameworks and methodologies that can effectively incorporate uncertainty into data management and integration processes. By doing so, organizations can enhance the accuracy and reliability of their data-driven decisions, ultimately leading to better outcomes. This paper explores various techniques and approaches to manage and integrate data with a focus on handling uncertainty, providing a comprehensive overview of the current state of research and practical applications in the field.
Types of Uncertainty in Data
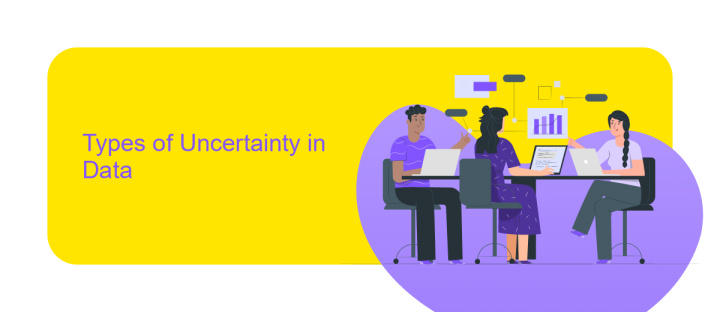
Uncertainty in data can arise from various sources, impacting the accuracy and reliability of data management and integration processes. One common type of uncertainty is measurement error, which occurs when data collected through sensors, surveys, or other methods contain inaccuracies due to limitations in the measurement instruments or human error. Another prevalent type is sampling error, which happens when a sample does not accurately represent the population from which it was drawn, leading to biased or incomplete data.
In the context of data integration, uncertainty can also emerge from semantic inconsistencies, where different data sources use varying terminologies or formats for the same information. This can be addressed by using integration services like ApiX-Drive, which facilitate the harmonization of data from multiple sources by automating the transformation and mapping processes. Additionally, missing data is a critical form of uncertainty, where incomplete datasets can lead to skewed analyses and conclusions. Proper handling and imputation techniques are essential to mitigate the impact of missing data on overall data quality.
Handling Uncertainty in Data Management and Integration
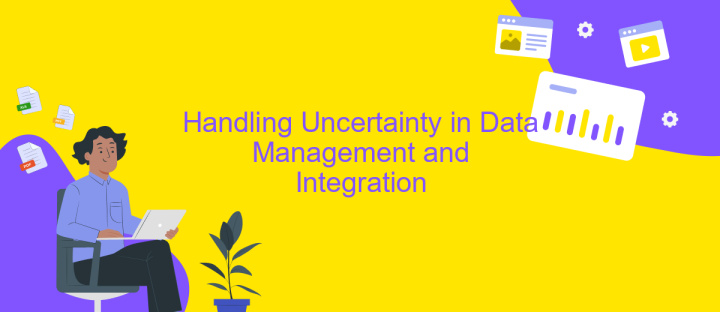
Handling uncertainty in data management and integration is crucial for achieving accurate and reliable results. Uncertainty can arise from various sources such as data quality issues, incomplete data, and inconsistencies across different data sources. Addressing these challenges requires a systematic approach to ensure data integrity and effective decision-making.
- Identify sources of uncertainty: Recognize where uncertainty originates, whether it is from data collection, processing, or integration stages.
- Implement data quality measures: Use techniques such as data validation, cleansing, and enrichment to improve data quality and reduce uncertainty.
- Utilize probabilistic methods: Apply statistical models and probabilistic algorithms to quantify and manage uncertainty in data.
- Integrate metadata: Maintain comprehensive metadata to provide context and traceability, helping to understand and mitigate uncertainty.
- Adopt robust data integration tools: Use advanced data integration platforms that can handle heterogeneous data sources and manage inconsistencies effectively.
By systematically addressing uncertainty in data management and integration, organizations can enhance the reliability of their data-driven insights. This proactive approach not only improves data quality but also supports better decision-making, ultimately leading to more successful outcomes in various applications.
Challenges and Limitations
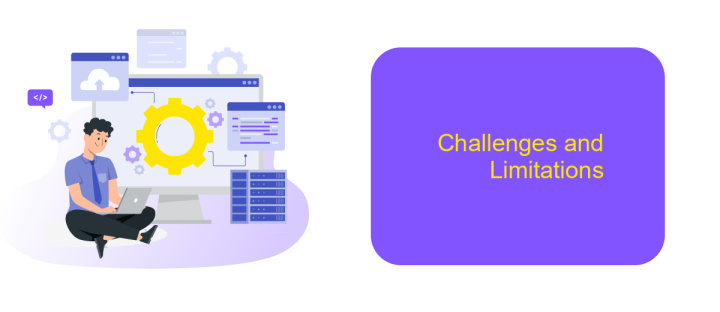
Incorporating uncertainty in data management and integration presents several challenges and limitations. One primary challenge is the inherent complexity of modeling and quantifying uncertainty, which often requires sophisticated statistical methods and computational resources. This complexity can lead to increased processing time and higher costs, making it difficult to implement at scale.
Another limitation is the potential for reduced data quality and reliability. When uncertainty is not properly managed, it can result in inaccurate or misleading insights, which can have significant consequences for decision-making processes. Additionally, integrating uncertain data from multiple sources can exacerbate these issues, as inconsistencies and discrepancies may arise.
- High computational cost and complexity
- Potential for reduced data quality and reliability
- Challenges in integrating data from multiple sources
- Difficulty in maintaining consistency and accuracy
Despite these challenges, addressing uncertainty in data management and integration is crucial for obtaining more robust and reliable insights. By developing advanced methods and tools to handle uncertainty, organizations can improve the accuracy and effectiveness of their data-driven decisions, ultimately leading to better outcomes.
- Automate the work of an online store or landing
- Empower through integration
- Don't spend money on programmers and integrators
- Save time by automating routine tasks
Conclusion
Incorporating uncertainty in data management and integration is a critical step towards enhancing the robustness and reliability of data-driven systems. By acknowledging and addressing the inherent uncertainties in data sources, organizations can make more informed decisions, reduce risks, and improve overall data quality. This approach not only helps in better prediction and analysis but also ensures that the data integration process is more resilient to inconsistencies and errors.
Tools like ApiX-Drive play a pivotal role in managing these uncertainties by providing seamless integration solutions that can adapt to varying data conditions. By automating data flows and offering real-time synchronization, ApiX-Drive helps organizations maintain data accuracy and consistency across multiple platforms. This ensures that even with the presence of uncertainties, the integrated data remains reliable and actionable, ultimately leading to more effective decision-making and operational efficiency.
FAQ
How does uncertainty affect data management and integration?
What are some common sources of uncertainty in data integration?
How can uncertainty be mitigated in data integration processes?
What tools can be used to automate data integration while managing uncertainty?
Why is it important to incorporate uncertainty management in data integration strategies?
Strive to take your business to the next level, achieve your goals faster and more efficiently? Apix-Drive is your reliable assistant for these tasks. An online service and application connector will help you automate key business processes and get rid of the routine. You and your employees will free up time for important core tasks. Try Apix-Drive features for free to see the effectiveness of the online connector for yourself.