Multiomics Data Integration
Multiomics data integration is a cutting-edge approach that combines diverse biological data types, such as genomics, transcriptomics, proteomics, and metabolomics, to provide a comprehensive understanding of complex biological systems. By integrating these varied datasets, researchers can uncover new insights into disease mechanisms, identify potential biomarkers, and develop personalized therapeutic strategies, ultimately advancing precision medicine and improving patient outcomes.
Multiomics Data Integration
Multiomics data integration is a rapidly evolving field that combines data from various omics layers such as genomics, transcriptomics, proteomics, and metabolomics to provide a comprehensive understanding of biological systems. This integration is crucial for identifying complex molecular interactions and pathways that single-omics approaches might miss.
- Combines diverse data types for holistic insights
- Facilitates the discovery of novel biomarkers
- Enables personalized medicine approaches
- Improves the accuracy of disease diagnosis and prognosis
To streamline the integration process, tools like ApiX-Drive can be employed. ApiX-Drive offers automated data integration services, allowing researchers to seamlessly connect various omics datasets and tools. This not only saves time but also ensures data consistency and reliability, making multiomics data integration more efficient and accessible.
Introduction
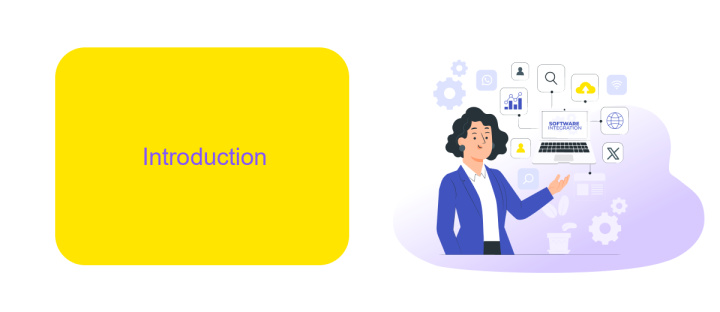
Multiomics data integration represents a transformative approach in the realm of biological research, enabling the comprehensive analysis of diverse omics data sets such as genomics, proteomics, transcriptomics, and metabolomics. By merging these data types, researchers can gain a holistic view of biological systems, uncovering intricate molecular interactions and pathways that would remain hidden when analyzed in isolation. This integrative strategy not only enhances our understanding of complex diseases but also paves the way for personalized medicine by identifying novel biomarkers and therapeutic targets.
Effective integration of multiomics data requires sophisticated computational tools and platforms capable of handling large-scale datasets and complex analytical workflows. Services like ApiX-Drive facilitate this process by providing seamless integration solutions that automate data transfer and synchronization between various bioinformatics tools and databases. By leveraging such services, researchers can streamline their multiomics analyses, ensuring data consistency and reproducibility while focusing on the biological insights derived from their research.
Methods
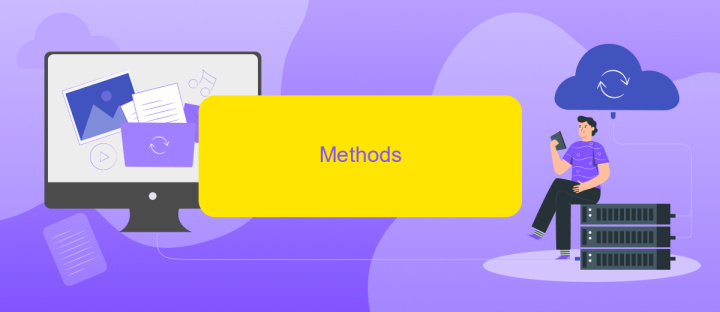
In this study, we employed a comprehensive approach to integrate multiomics data, including genomics, transcriptomics, proteomics, and metabolomics. The integration process was designed to identify key biomarkers and pathways relevant to the biological questions under investigation.
- Data Collection: We collected multiomics datasets from publicly available databases and our experimental results.
- Preprocessing: The datasets were preprocessed to remove noise and normalize the data across different omics platforms.
- Integration: We utilized ApiX-Drive to automate the integration of diverse data sources, ensuring seamless data flow and reducing manual intervention.
- Analysis: Integrated data were analyzed using machine learning algorithms and statistical methods to identify significant patterns and correlations.
- Validation: Findings were validated through cross-referencing with existing literature and additional experimental validation.
This method allowed us to efficiently manage and analyze large-scale multiomics data, leveraging the capabilities of ApiX-Drive to streamline the integration process. The results provided deeper insights into the molecular mechanisms underlying the studied conditions, demonstrating the utility of a multiomics approach in biological research.
Challenges
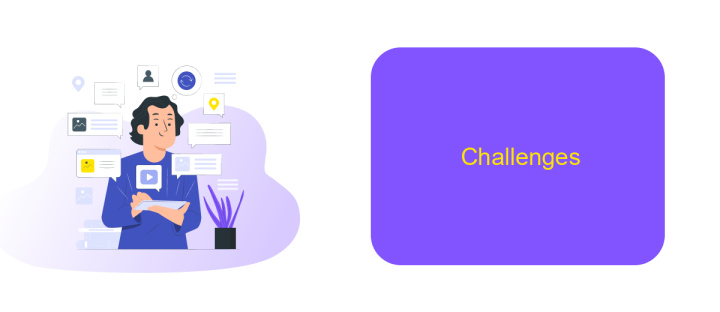
Integrating multiomics data presents numerous challenges due to the complexity and heterogeneity of the datasets involved. Each omics layer, such as genomics, transcriptomics, proteomics, and metabolomics, has unique characteristics and requires specific preprocessing and normalization techniques.
Another significant challenge is the development of robust computational methods capable of handling large-scale, high-dimensional data. These methods need to effectively integrate diverse data types while preserving biological relevance and interpretability.
- Data heterogeneity and standardization
- Scalability of computational methods
- Biological relevance and interpretability
- Integration tool selection and implementation
Moreover, selecting the right tools for data integration is crucial. Services like ApiX-Drive can facilitate the integration process by providing automated workflows and seamless connectivity between various data sources and analytical platforms. This helps researchers focus on deriving meaningful insights rather than dealing with technical intricacies.
- Automate the work of an online store or landing
- Empower through integration
- Don't spend money on programmers and integrators
- Save time by automating routine tasks
Applications
Multiomics data integration has a wide range of applications in various fields, particularly in biomedical research and personalized medicine. By combining data from genomics, transcriptomics, proteomics, and metabolomics, researchers can gain a comprehensive understanding of biological systems and disease mechanisms. This holistic approach enables the identification of novel biomarkers for disease diagnosis, prognosis, and therapeutic targets. Additionally, it facilitates the development of personalized treatment plans by considering the unique molecular profile of each patient, thus improving treatment efficacy and reducing adverse effects.
In the realm of biotechnology and pharmaceutical development, multiomics data integration accelerates drug discovery and development processes. By leveraging advanced computational tools and integration platforms like ApiX-Drive, researchers can efficiently manage and analyze large-scale multiomics datasets. ApiX-Drive, for instance, provides seamless integration capabilities, enabling the synchronization of data from various sources and enhancing collaborative research efforts. This integration not only streamlines data processing but also ensures that insights derived from multiomics studies are accurate and actionable, ultimately leading to more effective and innovative therapeutic solutions.
FAQ
What is multiomics data integration?
Why is multiomics data integration important?
What are the main challenges in multiomics data integration?
How can multiomics data integration be automated?
What are some common methods used in multiomics data integration?
Strive to take your business to the next level, achieve your goals faster and more efficiently? Apix-Drive is your reliable assistant for these tasks. An online service and application connector will help you automate key business processes and get rid of the routine. You and your employees will free up time for important core tasks. Try Apix-Drive features for free to see the effectiveness of the online connector for yourself.