Machine Learning Workflow Automation
In today's rapidly evolving technological landscape, automating the machine learning workflow has become essential for improving efficiency and scalability. From data preprocessing to model deployment, automation streamlines complex processes, reduces human error, and accelerates time-to-market. This article delves into the key components and benefits of machine learning workflow automation, providing insights on how to leverage these advancements for optimal performance and innovation.
Introduction
Machine Learning Workflow Automation has become a critical aspect of modern data science, enabling organizations to streamline their processes and enhance productivity. By automating repetitive tasks, data scientists can focus on more complex and creative aspects of their work, ultimately driving innovation and efficiency.
- Reduction of manual intervention, minimizing human error
- Improved scalability and consistency in model deployment
- Enhanced collaboration through standardized processes
Integrating various tools and services is essential for achieving seamless workflow automation. Services like ApiX-Drive facilitate these integrations by connecting different applications and automating data transfer between them. This ensures that workflows are not only automated but also interconnected, allowing for real-time data updates and streamlined operations. Adopting such solutions can significantly reduce the time and effort required to manage machine learning workflows, making it easier to maintain and scale them as needed.
Benefits of Machine Learning Workflow Automation
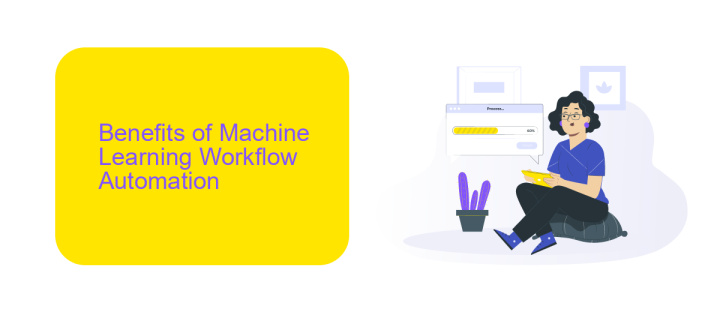
Machine learning workflow automation offers numerous benefits that significantly enhance the efficiency and effectiveness of data-driven projects. By automating repetitive and time-consuming tasks, such as data preprocessing, model training, and evaluation, teams can focus on more strategic aspects of their projects. This not only accelerates the development cycle but also reduces the likelihood of human error, ensuring higher quality outcomes. Additionally, automation facilitates better resource management by optimizing computational power and reducing operational costs.
Integration of various tools and services is another critical advantage of workflow automation. Services like ApiX-Drive enable seamless integration between different platforms, allowing for smooth data transfer and real-time updates. This ensures that all components of the machine learning pipeline are synchronized, enhancing collaboration and data accuracy. Furthermore, automated workflows provide scalability, making it easier to handle large datasets and complex models. As a result, businesses can achieve faster time-to-market and gain a competitive edge in their industry.
Challenges of Machine Learning Workflow Automation
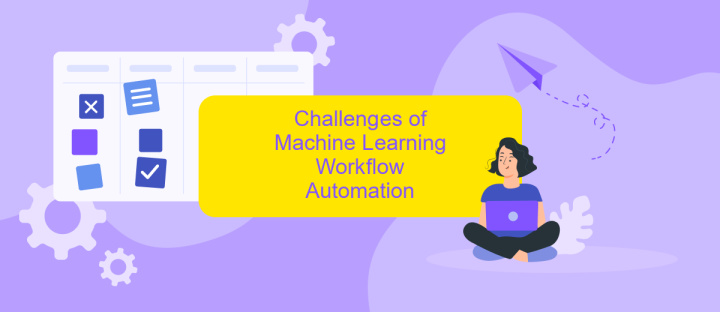
Automating the machine learning workflow presents several challenges that can hinder its effective implementation. These challenges range from technical complexities to operational hurdles, each requiring careful consideration and strategic planning.
- Data Quality and Integration: Ensuring high-quality data and seamless integration from various sources is crucial. Tools like ApiX-Drive can facilitate this by automating data transfers and integrations.
- Model Selection and Validation: Choosing the right model and validating its performance can be time-consuming and requires expertise. Automation tools must be adaptable to different models and validation techniques.
- Scalability: As the volume of data grows, maintaining the scalability of the workflow becomes a significant challenge. Automated workflows need to be robust enough to handle large-scale data processing.
- Monitoring and Maintenance: Continuous monitoring and maintenance of machine learning models are essential to ensure they remain effective over time. Automation should include mechanisms for regular updates and performance checks.
- Compliance and Security: Ensuring compliance with data protection regulations and maintaining data security is critical. Automated workflows must incorporate stringent security measures.
Addressing these challenges requires a combination of advanced tools, strategic planning, and continuous monitoring. By leveraging platforms like ApiX-Drive for data integration and focusing on robust model management, organizations can streamline their machine learning workflows effectively.
Best Practices for Machine Learning Workflow Automation
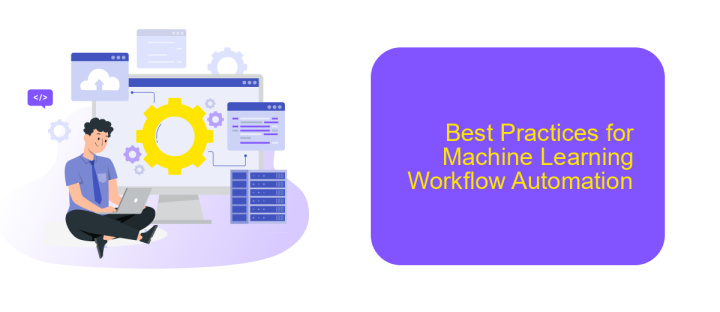
Automating your machine learning workflow can significantly enhance productivity and consistency. To achieve this, it's essential to follow best practices that ensure smooth and efficient operations.
First, focus on data preprocessing and cleaning. Automate the steps for data collection, transformation, and validation to ensure high-quality inputs for your models. This will save time and reduce the risk of errors.
- Implement version control for datasets and models.
- Use automated tools for hyperparameter tuning.
- Integrate continuous integration/continuous deployment (CI/CD) pipelines.
- Leverage cloud-based solutions for scalability.
- Ensure proper monitoring and logging of model performance.
Integrating various services can further streamline the process. For instance, using ApiX-Drive can facilitate seamless data integration across different platforms, automating data flow and synchronization. This allows you to focus more on model development and less on manual data handling, leading to more efficient and reliable machine learning workflows.
Conclusion
In conclusion, automating the machine learning workflow is essential for enhancing efficiency, reducing errors, and accelerating the deployment of models. By leveraging advanced tools and platforms, organizations can streamline the processes of data collection, preprocessing, model training, and evaluation. This not only saves time but also allows data scientists to focus on more strategic tasks, thereby driving innovation and achieving better outcomes.
Additionally, integrating various components of the workflow can be greatly simplified using services like ApiX-Drive. Such platforms enable seamless connections between different applications and data sources, ensuring a smooth and automated flow of information. As a result, businesses can achieve a higher degree of automation and scalability, ultimately leading to more robust and reliable machine learning solutions. Embracing these technologies is a critical step toward staying competitive in the rapidly evolving landscape of artificial intelligence.

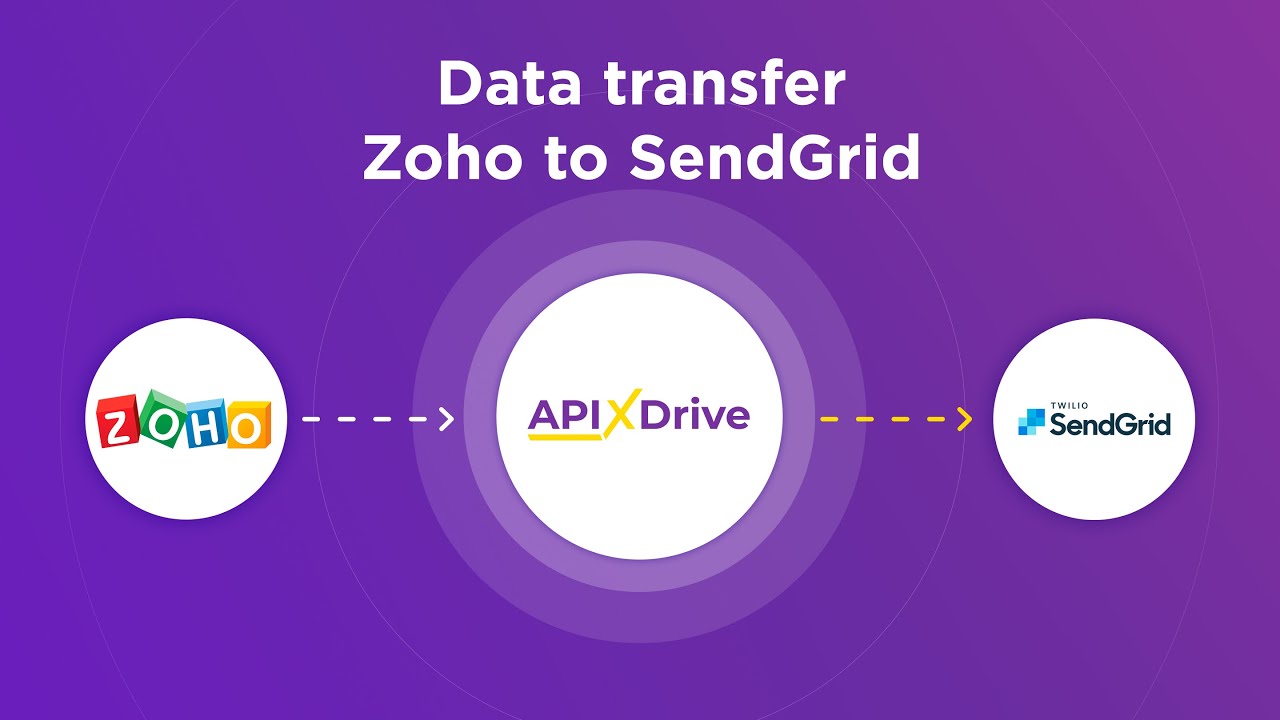
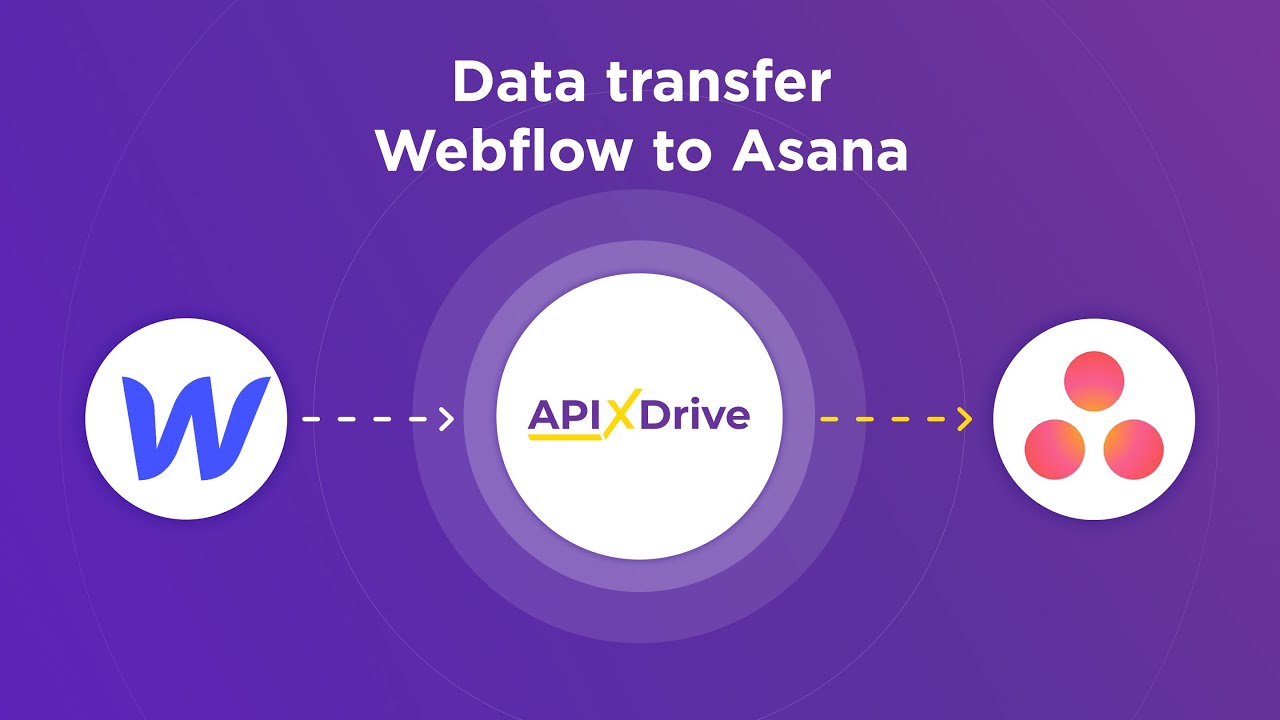
FAQ
What is Machine Learning Workflow Automation?
Why is automating machine learning workflows important?
What are the main components of a machine learning workflow that can be automated?
How can I integrate various tools and services for machine learning workflow automation?
What are the challenges in automating machine learning workflows?
Time is the most valuable resource in today's business realities. By eliminating the routine from work processes, you will get more opportunities to implement the most daring plans and ideas. Choose – you can continue to waste time, money and nerves on inefficient solutions, or you can use ApiX-Drive, automating work processes and achieving results with minimal investment of money, effort and human resources.