Multi-omics Data Integration, Interpretation, and Its Application
Multi-omics data integration represents a transformative approach in biological research, combining genomics, proteomics, transcriptomics, and other omics layers to provide a comprehensive view of cellular processes. This integrative method enhances our ability to interpret complex biological systems, uncovering novel insights into disease mechanisms and therapeutic targets. Its applications span across personalized medicine, biomarker discovery, and the advancement of precision healthcare.
Multi-omics Data Integration and Interpretation
Multi-omics data integration and interpretation involve combining diverse biological datasets to gain comprehensive insights into complex biological systems. This approach leverages data from genomics, transcriptomics, proteomics, metabolomics, and other omics technologies to provide a holistic view of cellular processes.
- Genomics: Study of an organism's complete set of DNA, including all of its genes.
- Transcriptomics: Analysis of RNA transcripts produced by the genome under specific circumstances.
- Proteomics: Examination of the entire set of proteins expressed by a genome, cell, tissue, or organism.
- Metabolomics: Study of chemical processes involving metabolites, providing a snapshot of the metabolic state.
Integrating these datasets enables researchers to uncover molecular mechanisms underlying diseases, identify biomarkers for diagnosis and prognosis, and develop targeted therapies. Advanced computational methods and machine learning algorithms are essential for managing and interpreting the vast amounts of data generated, facilitating the translation of multi-omics research into clinical applications.
Methods for Multi-omics Data Integration
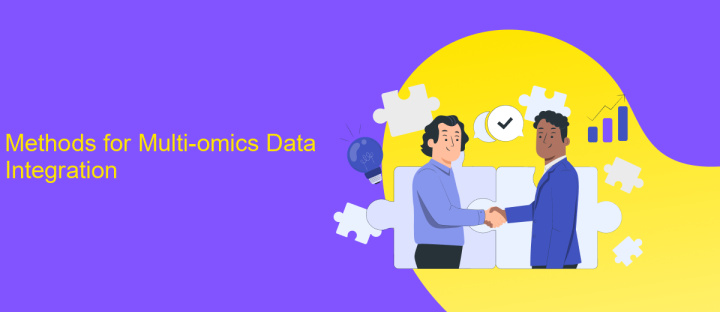
Multi-omics data integration involves the combination of datasets from various omics layers, such as genomics, transcriptomics, proteomics, and metabolomics, to provide a comprehensive understanding of biological systems. Methods for integrating these data include statistical approaches like principal component analysis (PCA) and canonical correlation analysis (CCA), as well as machine learning techniques such as random forests and deep learning. These methods help to identify patterns and correlations across different omics layers, facilitating the discovery of novel biomarkers and therapeutic targets.
In addition to traditional methods, modern tools and services like ApiX-Drive can streamline the integration process. ApiX-Drive offers automated data integration capabilities, allowing researchers to connect and synchronize multi-omics datasets from various sources effortlessly. This service supports the integration of diverse data types and formats, ensuring seamless data flow and reducing the complexity of manual data handling. By leveraging such tools, researchers can enhance the efficiency and accuracy of multi-omics data analysis, ultimately accelerating scientific discoveries and clinical applications.
Challenges and Opportunities in Multi-omics Data Analysis
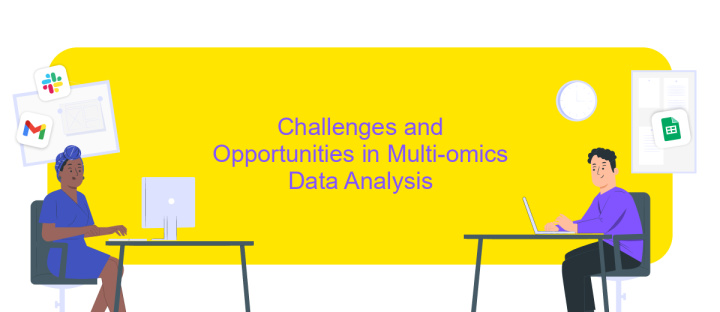
Multi-omics data analysis presents a unique set of challenges and opportunities for researchers. The integration of diverse omics data types, such as genomics, transcriptomics, proteomics, and metabolomics, can provide a more comprehensive understanding of biological systems. However, this integration is not without its difficulties.
- Data Heterogeneity: Different omics data types vary in their scale, format, and noise levels, making integration complex.
- Computational Complexity: Advanced algorithms and significant computational resources are required to process and analyze large-scale multi-omics data.
- Interpretation: The biological interpretation of integrated data remains challenging due to the complexity of underlying biological networks and pathways.
- Data Standardization: Lack of standardized protocols and formats for data collection and preprocessing can hinder effective integration.
- Scalability: As the volume of omics data grows, scalable methods for storage, processing, and analysis are essential.
Despite these challenges, multi-omics data analysis offers unparalleled opportunities for discovering novel biomarkers, understanding disease mechanisms, and developing personalized medicine approaches. By addressing these challenges through innovative solutions and collaborative efforts, the potential of multi-omics data can be fully realized, paving the way for significant advancements in biomedical research.
Machine Learning and Statistical Approaches for Multi-omics Integration
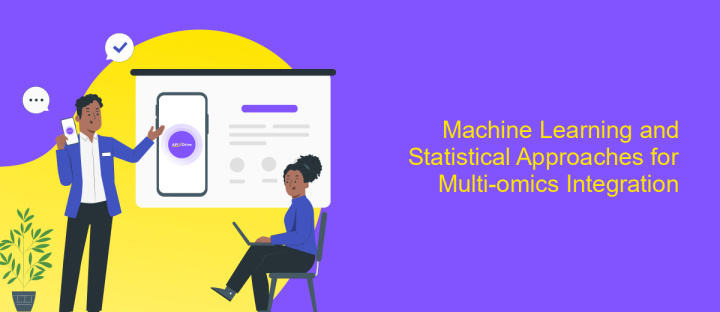
Machine learning and statistical approaches have become indispensable tools in the integration of multi-omics data. These methods enable the extraction of meaningful insights from complex biological datasets, facilitating a deeper understanding of underlying biological processes. By leveraging various algorithms, researchers can identify patterns and correlations that might not be apparent through traditional analysis techniques.
The integration of multi-omics data often involves several steps, including data preprocessing, feature selection, and model training. Each step requires careful consideration to ensure the accuracy and reliability of the results. Machine learning models, such as random forests, support vector machines, and neural networks, are frequently employed to handle the high-dimensionality and heterogeneity of omics data.
- Random forests: Useful for classification and regression tasks, capable of handling large datasets.
- Support vector machines: Effective for classification, especially in high-dimensional spaces.
- Neural networks: Powerful for capturing complex patterns and interactions within the data.
Statistical methods, including principal component analysis (PCA) and canonical correlation analysis (CCA), are also crucial for reducing dimensionality and identifying significant features. Combining these approaches provides a robust framework for multi-omics data integration, ultimately advancing our understanding of biological systems and improving the accuracy of predictive models.
Applications of Multi-omics Data in Personalized Medicine, Disease Diagnosis, and Drug Discovery
Multi-omics data integration has revolutionized personalized medicine by providing a comprehensive view of an individual's biological makeup. By combining genomics, proteomics, metabolomics, and other omics data, clinicians can tailor treatments to the specific genetic and molecular profile of each patient. This holistic approach enhances the precision of therapies, reduces adverse effects, and improves patient outcomes. Tools like ApiX-Drive facilitate the seamless integration of diverse omics datasets, enabling healthcare professionals to access and interpret complex biological information efficiently.
In disease diagnosis, multi-omics data offers unprecedented accuracy by identifying biomarkers that are indicative of specific diseases. This leads to earlier and more reliable diagnoses, allowing for timely intervention. In drug discovery, the integration of multi-omics data accelerates the identification of novel therapeutic targets and biomarkers, streamlining the development of new drugs. By leveraging platforms that integrate and analyze multi-omics data, researchers can uncover intricate biological pathways and interactions, paving the way for innovative treatments and personalized healthcare solutions.
FAQ
What is Multi-omics Data Integration?
Why is Multi-omics Data Integration important?
What are the common challenges in Multi-omics Data Integration?
How can automation services like ApiX-Drive assist in Multi-omics Data Integration?
What are some applications of Multi-omics Data Integration?
Apix-Drive is a simple and efficient system connector that will help you automate routine tasks and optimize business processes. You can save time and money, direct these resources to more important purposes. Test ApiX-Drive and make sure that this tool will relieve your employees and after 5 minutes of settings your business will start working faster.